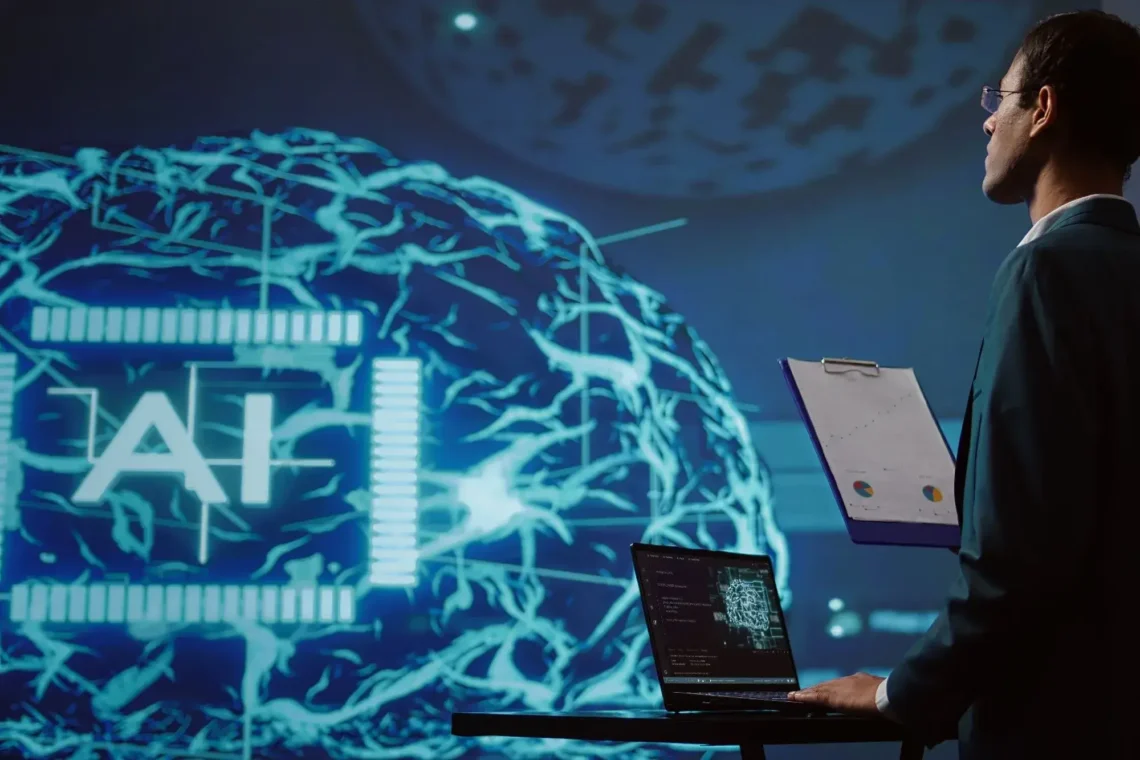
The Role of AI in Enhancing Fraud Detection in Banking
In today’s digital age, where online banking and financial transactions dominate the financial sector, fraud has unfortunately become a persistent issue. However, artificial intelligence (AI) is stepping up to become a game-changer in combating fraud in banking. With its ability to learn, predict, and analyze vast amounts of data, AI is revolutionizing how banks detect and prevent fraudulent activities. In this article, we will explore how AI enhances fraud detection in the banking industry and what the future holds for this powerful technology.
Fraud in the Banking Sector
Fraud in the banking sector encompasses a wide range of illicit activities, each targeting different vulnerabilities within financial systems. Common forms of fraud include identity theft, where fraudsters steal personal information to access accounts or open lines of credit. Phishing schemes are also rampant, with criminals using deceptive emails or messages to trick individuals into providing sensitive information. Credit card fraud, where unauthorized transactions are made using stolen card details, continues to be a major concern. Moreover, more advanced forms of cyber-attacks, such as money laundering, have evolved to exploit weaknesses in online banking and financial transactions. These fraudulent activities not only compromise the financial stability of customers but also place immense pressure on banks to improve their security frameworks.
The impact of fraud is felt on multiple levels. Customers who fall victim to fraud often suffer significant financial losses and emotional distress. For financial institutions, the consequences are equally severe, including monetary losses due to reimbursements, damage to their reputation, and the erosion of customer trust. Fraud detection systems are not just a legal or financial requirement; they are essential for maintaining the integrity of the banking system. With the increasing complexity of cyber-attacks, banks must adopt more advanced solutions to safeguard both themselves and their customers from these evolving threats.
The Evolution of Fraud Detection Methods
Fraud detection in banking has evolved significantly over time, moving from manual processes to more sophisticated technologies like artificial intelligence (AI). Early methods of fraud detection were slow and inefficient, relying heavily on human intervention and predefined rules. As fraud became more advanced, these traditional systems started to show their limitations, prompting the need for innovative solutions. The evolution of fraud detection has been a direct response to the growing complexity of cybercrime, as fraudsters continuously find new ways to exploit gaps in banking systems.
Traditional Methods of Fraud Detection
In the early stages of fraud detection, banks primarily used manual processes to spot suspicious activities. Bank employees would examine transactions, identify unusual patterns, and compare these to predefined rules or thresholds. These rules could be as simple as flagging transactions over a certain amount or those conducted in foreign countries. While effective to some extent, these methods were far from foolproof. They were labor-intensive, required significant human resources, and were prone to error due to the sheer volume of transactions processed daily. Banks could not keep up with the fast-paced and ever-changing techniques employed by fraudsters, resulting in many fraudulent activities slipping through the cracks.
However, one of the biggest drawbacks of these traditional methods was their reactive nature. Fraud would often only be detected after it had already occurred, leaving both banks and their customers vulnerable. Fraudulent activities could go unnoticed for extended periods, leading to significant financial losses before any corrective actions were taken. These traditional methods were simply not scalable, especially as the volume of digital transactions increased, and fraudsters became more sophisticated in their attacks.
The Limitations of Manual Processes
Despite their initial usefulness, manual fraud detection methods presented numerous limitations that hindered their effectiveness. One of the primary challenges was their reliance on static rules, which fraudsters could easily manipulate. For instance, if a bank had a rule that flagged transactions above $10,000, fraudsters would simply conduct multiple smaller transactions under that threshold to avoid detection. These static rules were often rigid and unable to adapt to the dynamic nature of fraudulent activities, rendering them less effective over time.
In addition to their inflexibility, manual processes were also slow and labor-intensive. With the growing number of financial transactions occurring daily, it became nearly impossible for human teams to review each one meticulously. As a result, many cases of fraud were either missed or detected too late. The lack of real-time monitoring also meant that corrective actions could only be taken after the fraud had already caused damage. Furthermore, the potential for human error made these systems unreliable in the long term, emphasizing the need for more automated and adaptive fraud detection methods.
Key limitations of traditional manual fraud detection:
- Static Rules – Unable to adapt to evolving fraud patterns.
- Time-Consuming – Slow process, not suited for real-time detection.
- High Resource Demand – Requires significant human oversight.
- Human Error – Prone to mistakes, especially with a high volume of transactions.
- Reactive, Not Proactive – Fraud is often detected after the damage is done.
The limitations of manual processes paved the way for the development of more advanced fraud detection techniques. As fraudsters began to exploit the shortcomings of traditional systems, banks started to adopt AI and machine learning to enhance their fraud detection capabilities. These technologies not only provide real-time monitoring but are also capable of learning from past data to predict and prevent fraudulent activities more effectively.
AI: A Game Changer for Fraud Detection
What is AI and How Does It Function in Fraud Detection
Artificial Intelligence (AI) is a groundbreaking technology that enables machines to simulate human intelligence by learning from data, identifying patterns, and making decisions. In the realm of fraud detection, AI plays a pivotal role by leveraging its ability to process vast quantities of data at lightning speed. Traditional systems would struggle to analyze the sheer volume of banking data generated every second, but AI algorithms can sift through both historical and real-time data to spot anomalies and irregularities indicative of fraudulent activity. By analyzing past patterns of behavior, AI systems are able to predict potential threats, enabling banks to stay one step ahead of fraudsters.
AI operates by training its algorithms on large datasets of past transactions, both legitimate and fraudulent. This training allows the AI to “learn” what a typical or unusual transaction looks like. The system can then apply this learning in real-time to incoming transactions, flagging those that deviate from expected norms. For instance, if a customer typically makes small purchases but suddenly tries to transfer a large sum of money to an overseas account, AI would recognize this as suspicious and alert the bank’s fraud team. AI’s ability to analyze and learn from massive data sets makes it an essential tool in modern banking fraud detection.
The Advantages of AI Over Traditional Methods
AI offers numerous advantages over traditional fraud detection systems, which were often based on predefined rules and manual intervention. One of the most significant benefits of AI is its ability to process enormous amounts of data in real-time, making it far more efficient than human operators or static rule-based systems. In traditional methods, human employees had to flag unusual activity based on rigid thresholds, but these methods often failed to account for the complexity and evolving nature of fraud. In contrast, AI systems can constantly monitor transactions as they occur, identifying suspicious activity with greater precision and speed.
Moreover, AI has the advantage of continuous learning. Traditional systems are static, meaning they operate based on predefined rules that fraudsters can easily manipulate. However, AI learns from each interaction and continuously improves its algorithms based on new data. This dynamic approach allows AI to adapt to new fraud tactics, staying ahead of fraudsters who might attempt to exploit new vulnerabilities. Additionally, AI reduces the risk of false positives, where legitimate transactions are incorrectly flagged as fraudulent, which can frustrate customers. By learning from patterns and refining its understanding of “normal” activity, AI enhances the accuracy of fraud detection.
Factor | AI in Fraud Detection | Traditional Fraud Detection |
Data Processing Capability | Real-time analysis of vast data | Slow, limited to manual review and static rules |
Learning and Adaptability | Continuously adapts and learns new patterns | Fixed, rule-based, cannot learn from new data |
Detection Speed | Instant detection of anomalies | Delayed detection, often reactive |
False Positives | Lower rates due to adaptive learning | Higher rates, due to rigid thresholds |
AI Techniques Used in Fraud Detection
Machine Learning Algorithms
Machine learning, a key component of AI, involves creating models that can learn from data without being explicitly programmed. In fraud detection, machine learning algorithms are trained on large datasets that contain examples of both legitimate and fraudulent transactions. By studying these examples, the system can identify patterns of behavior that are indicative of fraud. Once trained, the algorithm can apply its learning to new data, flagging any transactions that deviate from what it has learned to be typical behavior. Machine learning is particularly effective in recognizing complex patterns that might be missed by traditional rule-based systems.
For example, machine learning can identify subtle irregularities, such as a customer who usually shops in their hometown but suddenly makes multiple large purchases in a foreign country. The system can analyze such anomalies and determine the likelihood of fraud based on various factors, such as the customer’s history and the characteristics of the transactions. What sets machine learning apart is its ability to evolve—if fraudsters change their tactics, the algorithm will “learn” the new patterns over time and adjust accordingly, ensuring that the system remains effective in detecting fraud.
Natural Language Processing (NLP)
Natural Language Processing (NLP) is another powerful AI technique used in fraud detection. NLP is designed to understand and process human language, making it particularly useful in analyzing textual data such as emails, messages, or other forms of communication. In the context of fraud detection, NLP helps banks detect potential phishing scams, fraudulent communication, or attempts to deceive customers through fake messages. By scanning large volumes of emails, social media messages, or chat logs, NLP systems can identify suspicious content and flag it for further investigation.
Phishing attempts, where fraudsters try to trick customers into revealing sensitive information such as passwords or bank account details, can be detected through NLP by recognizing key patterns in language that are often associated with scams. For instance, phishing emails frequently use urgent or alarming language, urging customers to take immediate action. NLP systems are trained to recognize these linguistic markers and can flag suspicious communications before they reach customers, thus preventing potential fraud from taking place. NLP is particularly effective in fighting social engineering tactics, where fraudsters manipulate human emotions and behavior to commit fraud.

